Fisher's Exact Test
Sales leaders often have to make decisions based on limited data. Here’s a typical example. We want to see how good salespeople are at forecasting. Let’s say there are two salespeople and they each Commit three deals to close in the quarter.

Looks like Bob is bad at forecasting. Would you reward Amy and penalize Bob? Could that observed difference be due to randomness? One way to answer these questions is to do a statistical test of significance. And since this is a small sample size, you can use Fisher’s Exact Test. [1] (For larger samples there are alternatives.) Fisher's exact test determines if there are nonrandom associations between two categorical variables (won or not won in this case).
Use our Fisher’s Exact calculator here: https://login.funnelcast.com/fisher/.
(Detailed instructions on page.)
In this case, we can reduce the problem to the following table. And get a p value of 0.
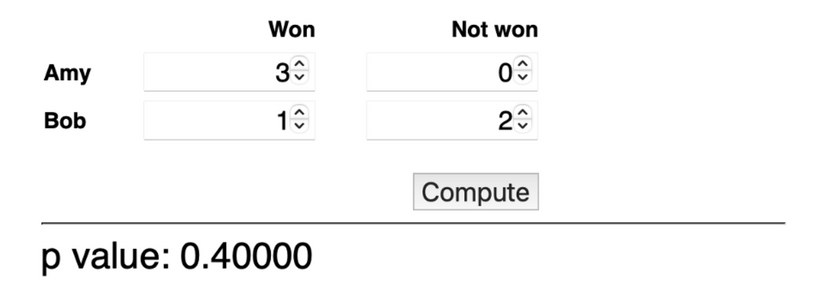
What’s that mean? [2] A p value of 0.4 means that there’s a 40% chance that the observed difference is due to random chance. That’s quite likely. Using the social-sciences 0.05 threshold [3], we can’t say that they are different at forecasting.
You can of course decide how confident you want to be about the decision. For instance, if you are ok being wrong about a decision 40% then go for it. Or, if that's all the data you have to go on, and you need to make a decision, then hope for the best knowing you will be wrong 40% of the time.
Note that this is just a test of whether Amy and Bob are performing differently. But let’s say that there is a 90% accuracy standard for Commits. Are Amy and Bob meeting the Commit standard?
You can use the Fisher’s Exact calculator to answer that question. In this case, we create an ideal predictor to compare Amy and Bob to. Something like 900,000 won, 100,000 not won. [4]
Doing this comparison, we find that Amy’s forecasting has a p value of 1.0000. It is indistinguishable from the ideal. Good for Amy.
But Bob’s forecasting has a p value of 0.02801. Using the 0.05 threshold, Bob is likely not meeting the standard.
It's very difficult to compare intuitively two points statistics. People are really bad at assessing probabilities. So for guidance on it, do a statistical test of significance. Use the Fisher's exact test when you have small data. [5]
Measurements presented without a sense of their variability are meaningless. You can't tell if they are really different from each other or even representative of the underlying process. You should seek a statistical significance test for every measurement you explore.
[1] Fisher's test assumes independence between Amy and Bob. Maybe that is so, maybe not.
[2] Statistical tests are based on a null hypothesis. In this case, the null hypothesis is that Amy and Bob are equally good at forecasting. The p value estimates how probable that is. The smaller the p value, the less likely is the null hypothesis. For sufficiently small p values, we reject the null hypothesis and conclude that there is a difference between Amy’s and Bob’s forecasting.
[3] In social sciences, the most common p value to reject the null hypothesis is 0.05. If the p value is less than 0.05 then there's a less than 5% chance that Amy and Bob are equally good at forecasting Commits.
[4] Those familiar with the Fisher's Exact formula may question the numerical stability of calculations based on such large figures. The calculator uses a numerically stable approximation.
[5] Small data is usually considered to be fewer than 10 observations per sample measurement. You can use other tests for larger samples, such as the Student's t-test.