How to quantify risk in your sales forecasts and plans.
A lot of basic sales data are not normally distributed. In our blog The Menace of Means, we explored what to do when summarizing these data. Non-normal distributions are very common in basic sales data. But sales forecasts are also sometimes non-normally distributed. That can wreak havoc on your ability to interpret and deliver on a forecast, unless you are appropriately aware.
Let's look at examples of how non-normal distributions arise and what to do about them. The examples here all assume forecasting for a set of existing opportunities for which the transaction values are known.
Traditional sales forecasts are made by making binary (include or exclude) judgement calls on deals. Answering the question for each deal, "will it close within the forecast period?" Add up the included deals to get the forecast. Sometimes there are two or more categories like “Commit” and “Upside.” We call these “classification” forecasts.
For very small sets of deals, this is a good way to forecast. In the extreme, if you had only one deal that can close in a forecast period, with a value of $100K you would either say the forecast is $0 or $100K. What you forecast depends on your confidence in the deal closing. And you would know that you have two possible outcomes. This is a bi-modal (very non-normal) distribution.
In contrast, “weighted” forecasts multiply every deal in your pipeline by its probability of closing in the forecast period. For instance, a $1M deal with 70% probability contributes $700K to the total forecast. (Even though you don’t win 70% of the deal.) This method is the mainstay of forecasting applications. The magic is in how an application determines probabilities.[1] For large sets of deals, this provides very good guidance.
One peril of weighted forecasts is that—for individual deals—they are always wrong. In the same way that the weather forecasts say "chance of rain today is 30%." On every day, that forecast will be wrong. It either rains or it doesn't. But, on average, it rains on 30% of days for which they make this claim. We accept this error because we live through many days. And on average, it will be helpful.
If you only had one deal to forecast, a $700K forecast for a $1M, 70% likely deal will always be wrong. A binary call will sometimes be right. But, with enough deals, the benefits of a weighted forecast shine.
This non-normal-distribution-of-outcomes problem is particularly noticeable for a small number of deals. But this same issue occurs with many deals, if you have high deal concentration. When a few large deals dominate the outcome, the average forecast can be misleading. There are several ways to assess and quantify this risk.
Pareto analysis
Concentration can be assessed with a Pareto chart. A Pareto chart shows the most significant factors contributing to an outcome. The 80/20 rule (80% of your business comes from 20% of your deals) is an example of a point on a pareto chart. Your business might be quite different.
Here is a sample pareto chart. The x-axis is the count-percentile of deal sizes—largest deals on the left to smallest on the right. The y-axis is the percent of total deal value. The curved line is the cumulative effect of all deals to the left of each point.
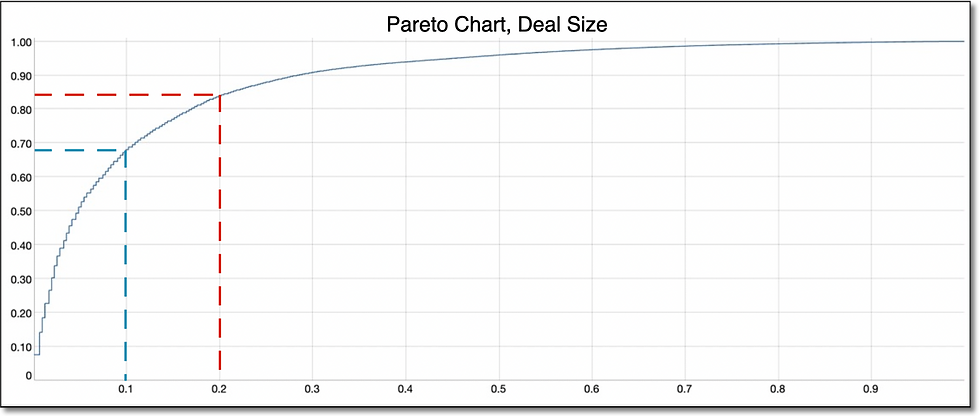
This business roughly follows the 80/20 rule (red lines). And 70% of the business comes from 10% of the deals (blue lines). If all deals were the same size, the chart would be a straight diagonal line from (0,0) to (1,1). This kind of analysis—run against open deals in your pipeline—will quickly point out the deal concentration risk in your business.
For this business, the first deal (the y-axis intercept) is about 7% of all deals. That’s a lot of deal concentration but we’ve seen situations where over 50% of the potential business is borne by a single deal.
See how to sell more. Try Funnelcast. |
Possible Outcomes/Prediction Density Chart
High deal concentration also shows up as a non-normal distribution in a histogram of possible outcomes. For better visualization of this, histograms are often smoothed and scaled. Statisticians call this a kernel density estimate. We call it a prediction density chart.
This chart below shows the distribution of possible outcomes for a set of deals. The bi-modal distribution is the result of uncertainty in the outcome of a single large deal. Many small deals make up the left-side hump. The right side hump is due to the one large deal and the smaller deals that can occur with it.

This is a great example of how weighted forecasts can be misleading. The mean outcome ($1.1M)—which is the weighted forecast prediction—almost never occurs because it is straddled by the two modes. Such are the perils of non-normal distributions and big deals in your pipeline.
This example above shows what can happen with one large deal. Below is a similar chart for a business with two large deals of different sizes. The distribution now has four modes reflecting different combinations of the two large deal outcomes. Most notably, yet again, the forecasted mean outcome (1.5M vertical line) almost never occurs.
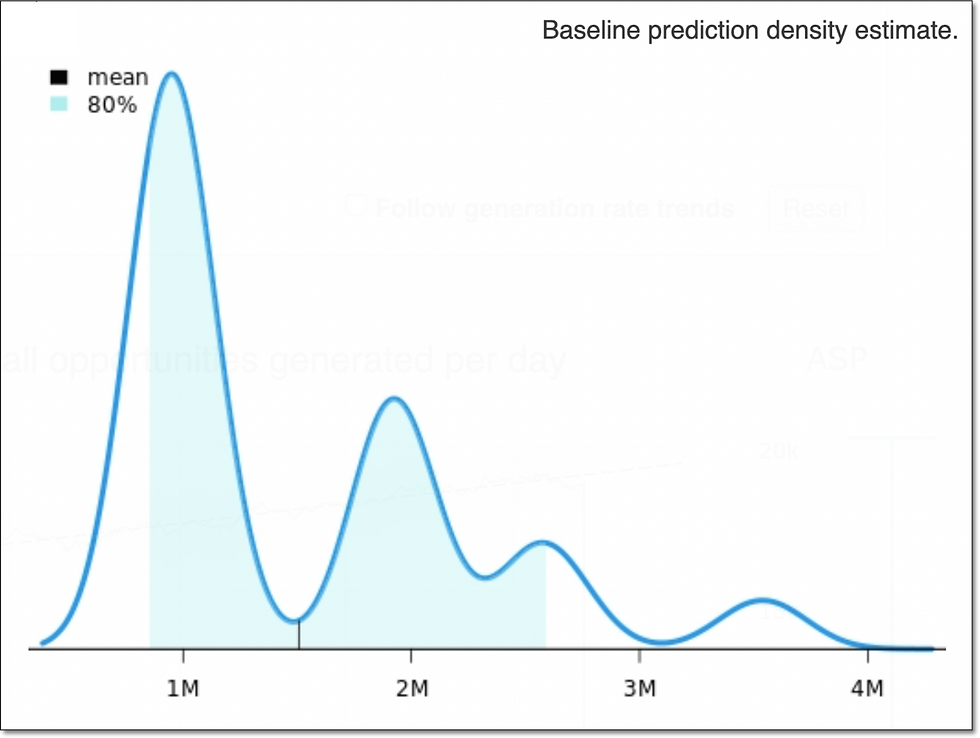
Isolate the Effects of Outliers
Another way to highlight the effect of big deals in your pipeline is to separately forecast the expected outcome due to these outlier deals. An historical forecast track shows for each day of the quarter, the contributions to the forecast from various sources: won deals (blues), prospective new funnel (green), open funnel normal sized deals (oranges), and open funnel big deals (red). This example was run mid way through the quarter. So, where the chart flattens out on the right side is the most recent forecast.
The size of the red area compared to the others is very important. It highlights the expected amount of the forecast related to big deals. Further inspection (not shown) highlights the specific deals that contribute to the red area. Toggling big deals off and on allows sales leaders to assess the effects of not closing any of them.
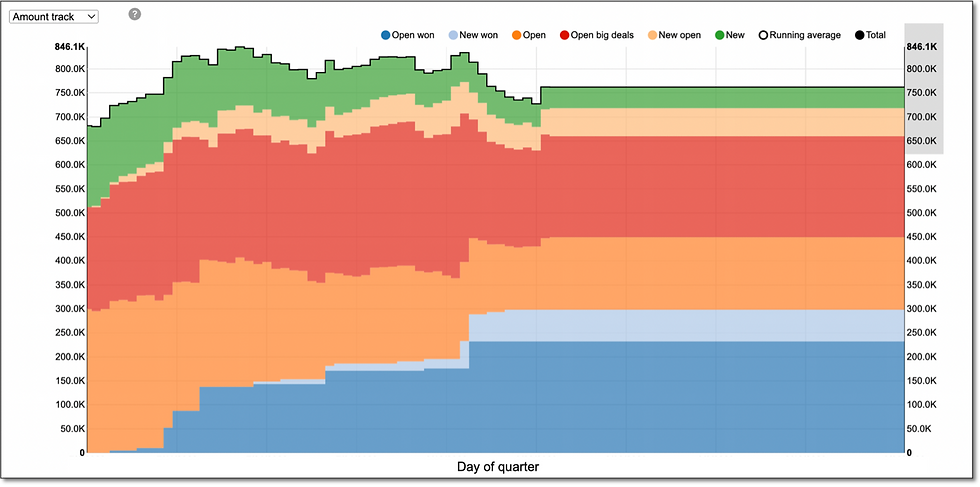
In this case the big deals for this quarter make up about half of the remaining forecast to be closed. That's real business risk that would be buried in an average statistic.
Deal concentration in your forecast is an important business risk to understand and quantify. Where large deals dominate the outcome, there are several ways to assess and quantify this risk. Funnelcast supports the three ways discussed here.
[1] Human assigned probabilities are often pretty good as well—if you clearly define that probability means the likelihood to close in the forecast period.
Komen