"Big Data" has become a ubiquitous buzzword in nearly every industry that captures and leverages data to inform operational and strategic decision making. The common sentiment is that large volumes of data, and analyzing all sorts of data are table stakes. Organizations that don’t capture “big data” internally, or lack the budget to pay for external data, are somehow handicapped.
In this blog post, we both explore and explode that myth—as it applies to B2B sales forecasting.
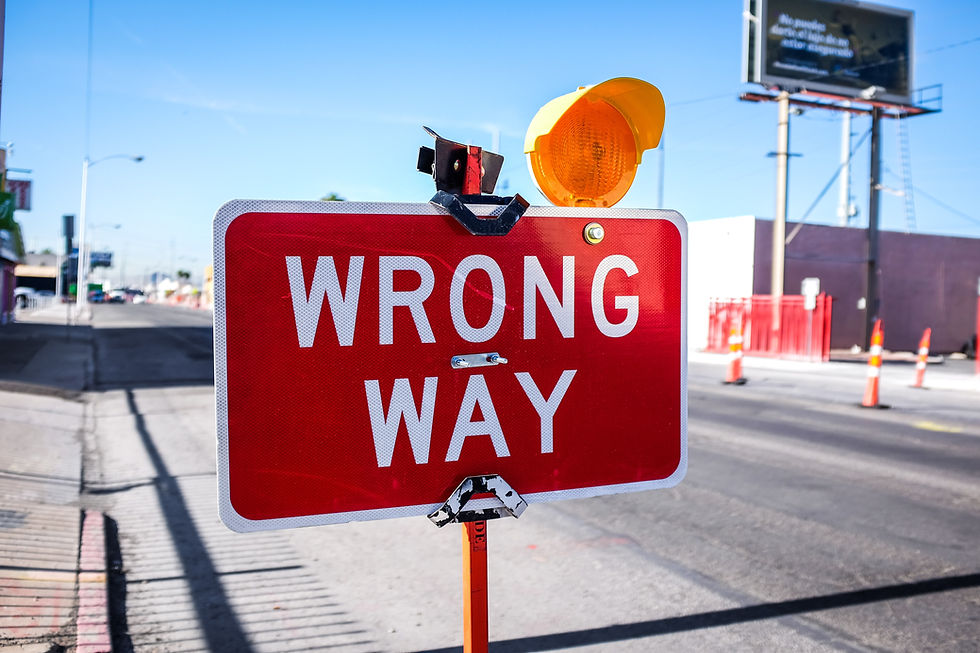
The data required for successful sales forecasting can be visualized as a cube—the axes of which are breadth, quality, and depth. Breadth means how many data fields containing predictive information are available. Quality refers to the accuracy, completeness and consistency of the data you’re capturing. Depth is about how much longitudinal history you have available in your model training period.
When it comes to sales forecasting, what matters most is depth, depth, and depth. Accuracy is nice but no one (and this includes you) has perfect data; so stop worrying about it. As to breadth… it’s a red herring. Less is often more.
To build reliable sales forecasts, we find that what really matters is the information content of the data that’s available. And that translates to having enough depth (data in your model training period) to capture the performance of a sales engine and to make up for the inevitable quality issues. Quality (accuracy) helps this as well of course, but there is a tradeoff between depth and quality. What you lack in quality, you can make up for in depth (read our post about that).
Notice that we didn’t mention breadth (lots of data fields). That’s because it’s the least important of the three—if you have a model you are imposing on the data. This is what the "AI" forecasting vendors all do. They call it AI, but it isn't. they are imposing a model on the data. If you are trying to use AI to develop a model, then a breadth and depth become more important. But for B2B sales forecasting applications that dataset is far larger than most businesses have available.
Once you have the core data elements needed to build a model, adding more data elements is actually a trap that leads to delayed implementation, and more complex models that take longer to compute, are harder to understand, and in many cases hurt the model’s performance.
Occam's Razor wins in this case. Adding more data breadth, especially redundant or highly correlated data often decreases forecast accuracy, and adds unnecessary training overhead as algorithms try to make sense of the messy “Breadth of Data” you’ve thrown into the decision making machine. The best model is the simplest model that explains the observed behavior.
See how to sell more. Try Funnelcast. |
The Funnelcast model requires just six standard data fields (Opportunity ID, Created date, Modified date, Close date, Amount, and one of the following: Stage, Forecast Category, Probability) to create accurate and timely sales forecasts. Here’s an example showing what you can accomplish with those six fields—a 365-day sliding window forecast backtest (comparing predictions to actual results).
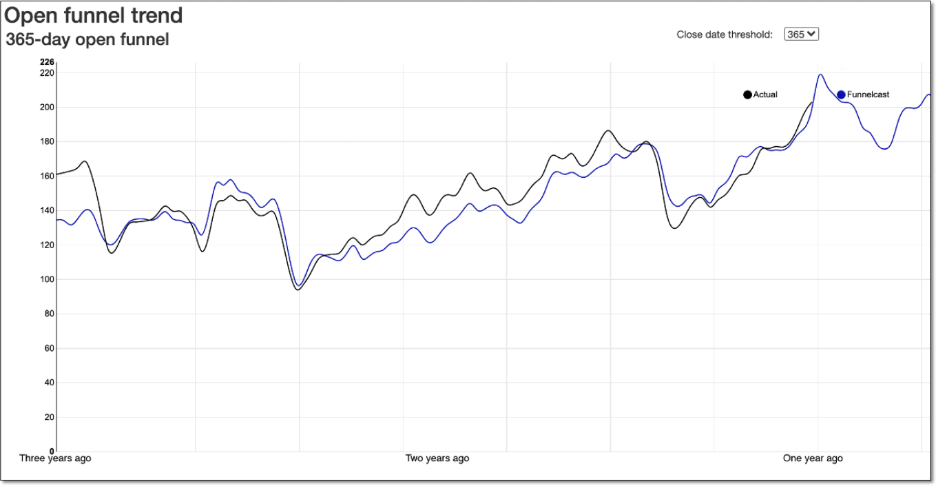
The blue line is the Funnelcast 365-day forward (sliding window) forecast—one each day over three years. It represents Funnelcast's prediction of what the business can expect over the next one-year period in closed business (in this case measured in new customer wins, it can be shown in revenue as well) from their then-current open funnel. The black line represents what happened over the same one-year period.
That’s a pretty good forecast—considering this is a year in advance of the actual results. Admittedly, they are not all that good. It was cherry-picked for marketing purposes. (What did you expect?) But let's underscore that (1) this is real sales data, not a synthetic make-believe dataset, and (2) there is really nowhere to hide to make the forecast look better. If you make enough forecasts, then you can always find one that was close to right. This is not one, but 730 forecasts (made over a two-year period) that are all pretty good. Now we are not saying that there is no value to using other fields. But how much better do you expect to get? These are one-year ahead forecasts.
There’s a reason we use these six fields—they are the most common fields used to track sales opportunity progression. And all CRM systems record these fields. Better yet, if you are using Salesforce, you are set; because by default, Salesforce automatically stores these fields and all their history for you.
While there are limits to such a simple model, it enables near instant deployment. Because the data are readily available, within minutes of connecting to your CRM data, Funnelcast can create accurate short- and long-term forecasts (like the one above), and powerful sales productivity insights (like the one below) that help you optimize your resources so you can sell more.
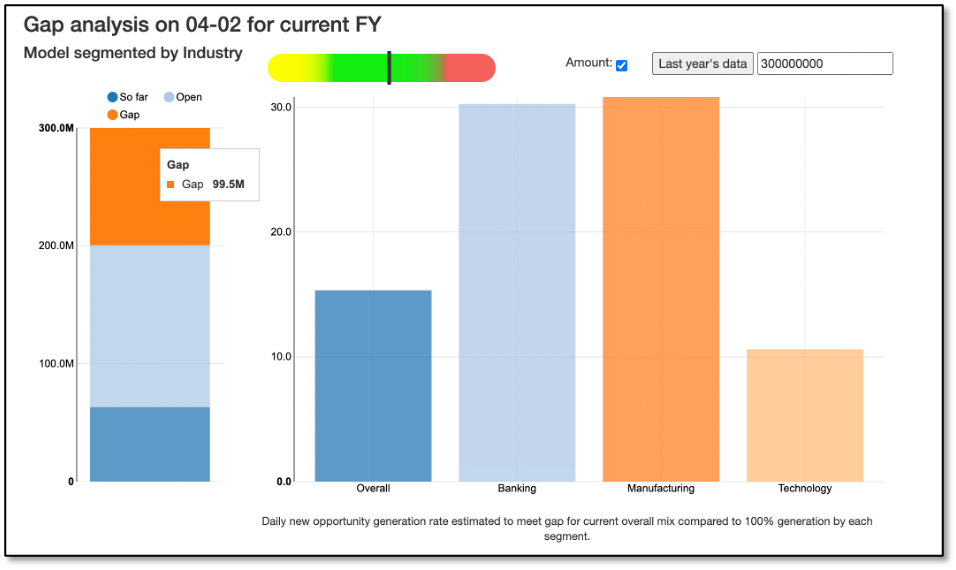
Gap analysis above shows how much of your plan you have closed to date (left, dark blue), what you can expect from your open funnel (left, light blue), and the gap to your plan (left, orange). When the analysis is segmented as here by Industry, the right side shows the rate of opportunity creation needed to meet the goal. In this case sales in the Technology sector are considerably more productive than in banking and manufacturing. This business could sell more by just focusing on Technology opportunities.
There are other signals that can improve a forecast. For example, you could scrape your website logs to find activity from a prospect or an account, you could employ an AI engine to read emails or interpret recorded conversations to pick out sentiment, or you can scour calendars to find activity signals that are not already recorded in your CRM system. And Funnelcast can use these fields—if you have them.
While all these signals may add predictive power to your forecasts, the benefit is at the margin. The cost and relevance of those signals needs close examination – what’s the quality of these signals and at what point in the sales cycle will they add predictive value? Typically, these signals are noisy (tech speak for poor quality data). Moreover, these other signals are strongest and most useful at the end of a sales cycle—where your sales management already knows what to expect. So, are these additional signals going to improve your forecasts enough to merit the cost and time of getting the data? Should you delay getting the benefit of CRM analytics to get this marginal improvement?
Our view? If you have this data today, use it. If you have other reasons to get that data, go for it. Throw it our way when you have it and we will see what predictive value it adds. But don’t delay your forecasting and sales optimization efforts. If you don’t have this data and have no plans for them... Don’t sweat it.
Comentarios