Just define what you mean by probability; your forecasts will improve, and you can get most of what you get from "AI" forecasting engines.
B2B companies often predict sales using a “classification forecast” in which each opportunity is classified in one of several categories. CRM systems provide a field for this. In Salesforce it’s called Forecast Category. The default values are: Commit, Best Case, Pipeline, Omit, or Closed. The forecast for the quarter consists of all won opportunities plus those in the Commit category; add in Best Case opportunities to show upside possibility. The strength of these classification forecasts is that they are easy to understand. But they are typically only accurate at the end of a sales cycle or quarter.
The figure below is an example from Funnelcast. It shows the daily evolution over the course of a quarter of two classification forecasts (Commit + won: bottom black line; and Best Case + Commit + won: top purple line). The stacked blue areas represent won sales: dark blue, sales from the open funnel at the start of the quarter; light blue, sales from new activity added after the start of the quarter. The thin, black line at the top of the stacked colored areas is the Funnelcast prediction. The colored areas represent different components of the Funnelcast forecast.
A perfect forecast would be a horizontal line ending exactly at the top of the right edge of the blue areas.
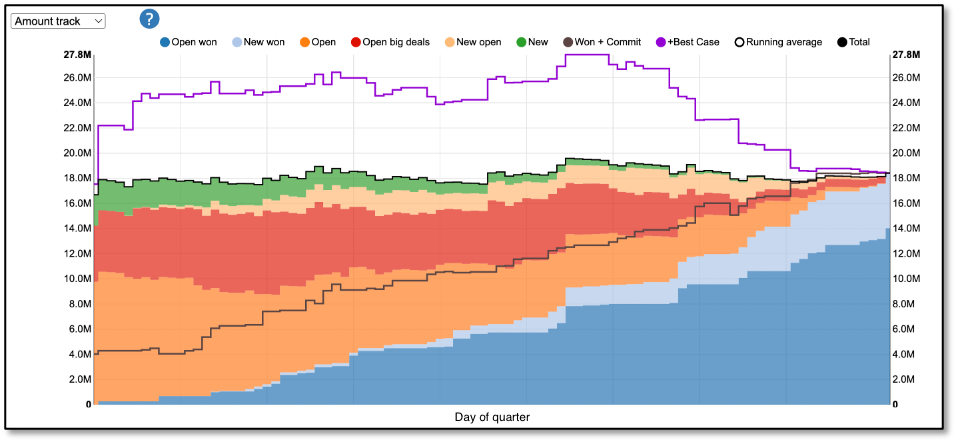
Those classification forecasts are pretty unhelpful... until the end of the quarter. Early in the quarter, the Commit is too conservative, and the Best Case is too optimistic.
For these reasons businesses turn to using a “weighted sales forecast” in which each individual opportunity amount is multiplied by its probability and then the total forecast is the sum of the individual weighted opportunities. The probability is either manually set, or scored by an "AI" sales forecasting engine.
See how to sell more. Try Funnelcast. |
Google “weighted forecast” and you will find many posts advocating this approach. You will even find people advocating to not do this because—as they rightfully point out—for small cohorts of opportunities, this can be very misleading. Consider a forecast consisting of one $100,000 opportunity with a 50% probability. The weighted forecast is $50,000, while the actual outcome will either be zero or $100,000. The forecast is guaranteed to be wrong.
But, for a large portfolio of opportunities, the weighted forecast is more accurate than a classification forecast—if you accept that for every individual opportunity, the forecast will be wrong. This is the tradeoff you make with a weighted forecast. Overall accuracy improves at the expense (perhaps) of the accuracy in picking out individual opportunities. It also gives you a much earlier view of what to expect.
Building a weighted forecast is simple. Every CRM system provides a probability field for this purpose and a derived field (or you can create one) representing the “expected” revenue (the product of the probability and the amount). A weighted forecast is just a summary report on that “expected” amount. But your forecast will only be as good as your probabilities.
To address this, forecasting vendors offer "AI" products that use statistical models to produce a probability for each opportunity. These products ease the forecasting burden and produce helpful scores for forecasting and setting priorities about what to work on. The models used for this are based on the probability score assigned by the salesperson along with other information like close date, sales stage, age, forecast category, activity, and a variety of other information sources such as calendars and emails.
Many of these products are marketed as “artificial intelligence” engines. They synthesize all this information to tell you what you should already know. Their forecasts are still useful however, because they quickly do at scale what is very time consuming for a human, thereby freeing time for selling and account reviews.
But here’s the rub; an analytic forecast is only as good as the data you feed it. All those fields feeding the “AI forecasting” engine are there to refine the probability. And there is one glaring imprecision in the meaning of probability you are feeding it. Before you invest in one of these products you should fix this so you can improve your forecasts, and maybe even entirely avoid the need to purchase a forecasting application.
What does it mean to say that we think an opportunity has a 50% chance of closing? By when does the 50% likelihood apply? By the indicated close date? The end of the current quarter? Ever?
If you want to use a weighted forecast to improve accuracy and get an earlier view of what to expect, then you should define precisely what probability means. We recommend using two different fields: one for the probability that an opportunity will ever be won and another for the probability that an opportunity will be won this quarter.
Use the standard Salesforce probability field for the “ever won” likelihood because it is automatically linked to sales stage. Salespeople can manually override this probability if the auto-assigned probability does not match their judgement. Create (and track the history of) a custom field called “CQ Probability” for the probability that an opportunity will be won in the current quarter. This should be a mandatory field if the Close Date is in the current quarter and should also be populated if there is any possibility of pulling the deal into the current quarter. You can then produce a weighted forecast as a simple report in Salesforce.
Making this one change and applying it consistently will improve the accuracy of weighted forecasts. If you are contemplating purchasing or already using a forecasting application, it will also improve the accuracy of those predictions. But you might even find that you don’t need a forecasting application.
If you have not yet made these changes, there is still a simple way to improve your weighted forecasts—without adding the new field. Read about that in part 2 of this post.
Comments